
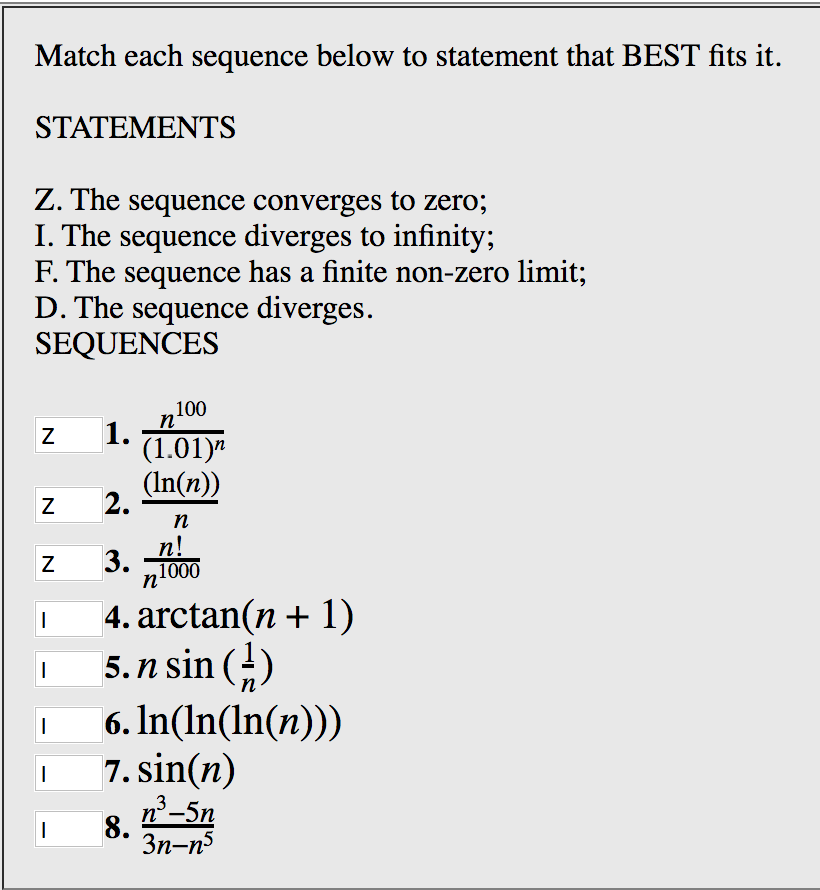
The actual required compute in this case is as follows: 9-mult 8-add ( nrows x (n-1)_cols) operations, only to throw away most of the computed results since they would be 0s (where we have pads). Thus, we will have to perform 6x9 = 54 multiplication and 6x8 = 48 addition Because there is still one pressing problem, mainly in terms of how much compute do we have to do when compared to the actually required computations.įor the sake of understanding, let's also assume that we will matrix multiply the above padded_batch_of_sequences of shape (6, 9) with a weight matrix W of shape (9, 3). So, the data preparation work should be complete by now, right? Not really.
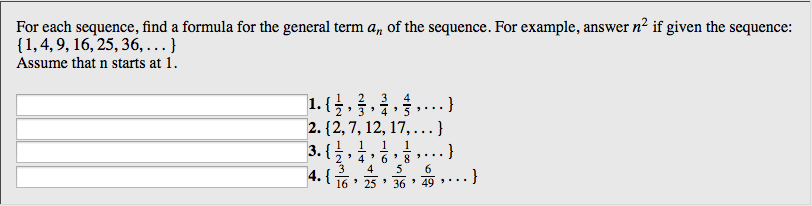
To do so, we have to pad all of the sequences (typically with 0s) in our batch to the maximum sequence length in our batch ( max(sequence_lengths)), which in the below figure is 9. Now, we want to pass these sequences to some recurrent neural network architecture(s). (The batch_size will vary depending on the length of the sequence (cf. You can also consider this number 6 as the batch_size hyperparameter. Let's assume we have 6 sequences (of variable lengths) in total. Consequently, the time required for training neural network models is also (drastically) reduced, especially when carried out on very large (a.k.a. TL DR: It is performed primarily to save compute. Here are some visual explanations 1 that might help to develop better intuition for the functionality of pack_padded_sequence(). >PackedSequence(data=tensor(), batch_sizes=tensor()) Torch.nn._padded_sequence(b, batch_first=True, lengths=)
#QUICKLENS EACH SEQUENCE CODE#
Here's a code example: a = ), torch.tensor()]ī = torch.nn._sequence(a, batch_first=True) I might have been unclear at some points, so let me know and I can add more explanations. This has been pointed by This can be passed to RNN and it will internally optimize the computations. This is helpful in recovering the actual sequences as well as telling RNN what is the batch size at each time step. Elements are interleaved by time steps (see example below) and other contains the size of each sequence the batch size at each step. Instead, PyTorch allows us to pack the sequence, internally packed sequence is a tuple of two lists. Moreover, if you wanted to do something fancy like using a bidirectional-RNN, it would be harder to do batch computations just by padding and you might end up doing more computations than required. You would end up doing 64 computations (8x8), but you needed to do only 45 computations. For example: if the length of sequences in a size 8 batch is, you will pad all the sequences and that will result in 8 sequences of length 8. When training RNN (LSTM or GRU or vanilla-RNN), it is difficult to batch the variable length sequences.

#QUICKLENS EACH SEQUENCE HOW TO#

_ case class Street (name : String ) case class Address (street : Option ) case class Person (addresses : List ) val person = Person (List (Īddress (Some (Street ( "1 Functional Rd." ) ) ),Īddress (Some (Street ( "2 Imperative Dr." ) ) ) ) ) val newPerson = modify (person ) ( _.
